From PhD research to real-world solutions: Interview with Frederique van Leeuwen
Posted on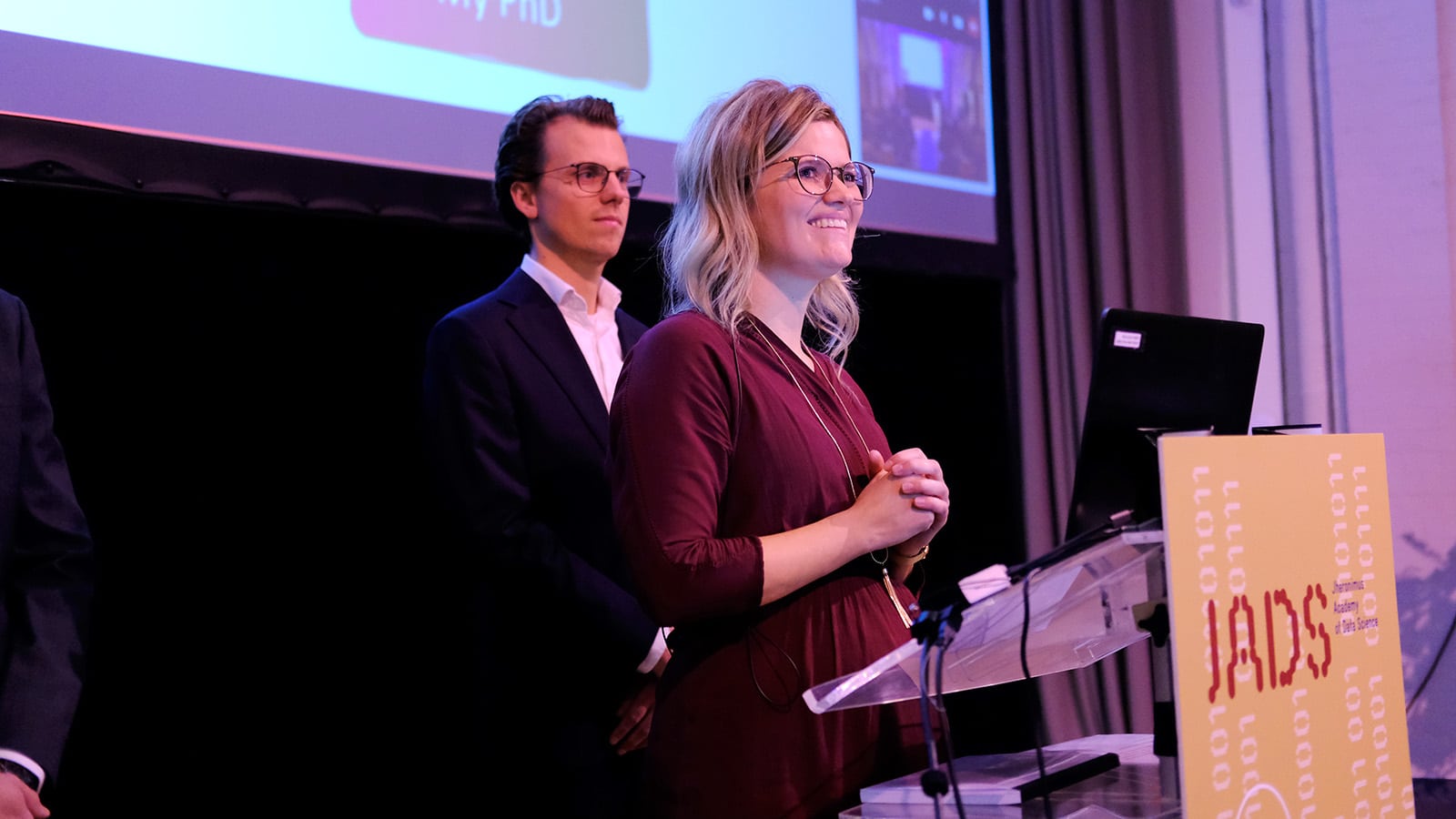
In our ever-connected world, where devices and sensors continuously exchange information, a vast amount of data is generated on a daily basis. Amidst this ocean of data lies a challenge: how can we turn all this data into meaningful knowledge? Frederique van Leeuwen, a committed PhD candidate at JADS, has taken on this challenge in her research.
Even though humans have a natural capacity to turn data into valuable knowledge, the volume, velocity and variety of incoming data streams make it impossible to exploit this capability. In contrast, computers contain exceptional processing power, allowing for complex calculations to be performed continuously.
The purpose of time series data mining is to derive meaningful insights and knowledge from time-dependent data. Identifying previously unknown, frequently occurring patterns in time series, known as motifs, helps to uncover hidden temporal structures and relationships within the data, and can serve as a subroutine for various time series data mining tasks across diverse application domains.
We had a conversation with Frederique where we explored the motivations behind her journey into time series motif discovery, aiming to understand the impact of her findings on the way we engage with the data around us.
Why did you choose this research subject and what makes it so fascinating?
“When I started my PhD journey, the research subject wasn’t well-defined yet. Close collaboration with several companies across diverse application areas highlighted the challenge of extracting meaningful knowledge from the shape of time series data. Motif discovery emerged as a useful tool to understand the underlying dynamics of time series behaviors, and its potential to address real-world challenges intrigued me.”
Which challenges did you meet along the way and how did you overcome them?
“Starting as one of the first PhD students at JADS was an incredible adventure filled with challenges. As everything was new for everyone, there often did not exist straightforward solutions to what normally might be minor challenges under different circumstances. Nevertheless, with a lot of patience and the generous help from numerous individuals (thank you!), I discovered that finding one’s way is an interesting journey of its own.”
What is the impact of your work in the real world?
“Motif discovery serves as a key ingredient in understanding many real-world challenges. However, extensive experiments revealed that commonly held assumptions about preprocessing steps can create a gap between the theory and the practical use of motif discovery. Building on these insights, we presented two practical use cases in the domains of time series labeling and driver distraction detection. For the latter, we conducted a naturalistic driving behavior experiment, gathering a substantial amount of time series data from the Controller Area Network (CAN) bus of cars. Our findings demonstrate that motif discovery can detect driver distraction with high precision, offering a means for insurance companies to shift from risk coverage to proactive risk reduction. This not only helps reduce costs but also may contribute to an overall safer driving environment by encouraging responsible driving behavior.”
What are your plans after your PhD?
“Working remotely during the pandemic provided me the opportunity to move to the north of Spain. Living in a little village surrounded by mountains, in combination with a freelance job as data science/optimization consultant was never planned, but turned out to be amazing. So, right now, I’m not actively seeking new plans or to change anything. I’ll just go with the flow and see what new adventure crosses my path.”