Column: Understandable AI-models
Posted on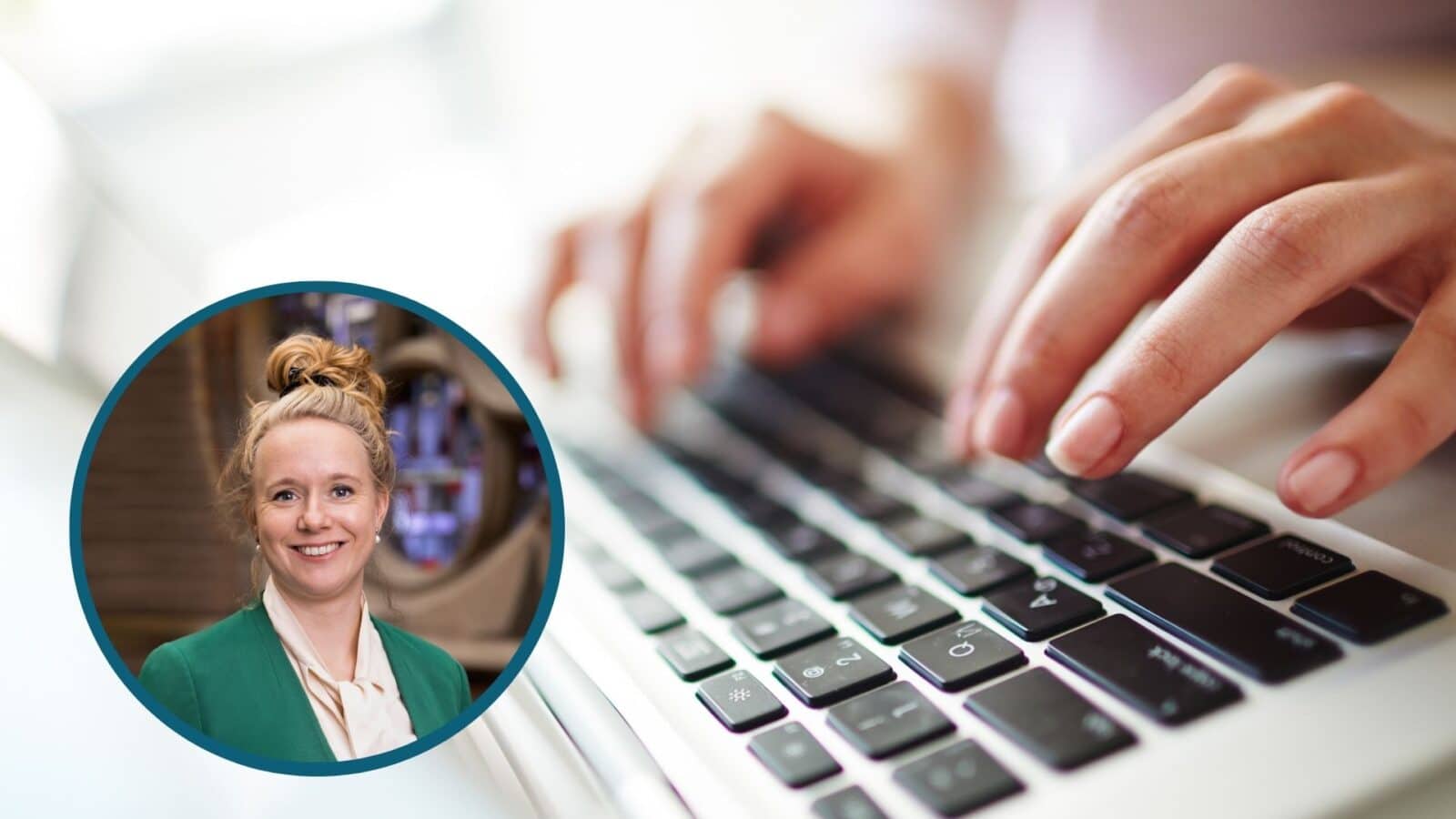
This column, written by Sandra van den Poll, partnership manager at JADS, was published before in the Food+Agribusiness magazine.
The advent of Generative AI has made the uses of algorithms in our daily lives much more visible. Texts can be written in the blink of an eye and even photos and videos can be created in a single click without any human intervention. The rapid development of Generative AI has also reached the agri-food sector. Algorithms are being used to optimize crops, detect diseases and make supply chains more efficient. While these developments are promising, it is crucial that these algorithms are and remain easy to understand.
Trust in AI is crucial. Business owners, consumers and regulators alike need to be able to understand how AI decisions are made. The quality of the data used to train AI models is key here. Incomplete or biased data can lead to incorrect results.
Moreover, it is crucial to be aware of any bias (a conscious or unconscious bias) in the model in order to understand the outcomes. First, there is a risk of bias due to the historical data set. If the data are mainly from large, traditional farms, then the algorithm may develop a bias in favor of these farms and their specific cultivation methods. As a result, the algorithm may be less accurate for smaller, more diverse farms. Other types of bias in a model can be caused by seasonal data, geographically specific data, as well as social bias. The latter category has been the subject of much controversy recently with regard to training AI models based primarily on Western social standards, which sometimes caused these models to produce discriminatory results for other social groups.
To avoid bias, we need to assure diverse and representative data sets. In addition, algorithms should be evaluated regularly and results should be transparent. By taking these measures, we can ensure that AI in agrifood remains fair and reliable.